세계에서 가장 강력한 AI를 만들겠다는 엘론 머스크의 야심 찬 프로젝트, 그록 3(Grok 3)가 AI 업계에 큰 화제를 불러일으키고 있습니다. 2025년 2월 17일에 출시된 이 다중모달 대규모 언어 모델은 무려 2.7조 개의 매개변수를 갖추고 있어 역대 가장 큰 AI 모델 중 하나로 평가됩니다7. 엘론 머스크는 이 모델이 "모든 기존 AI 시스템을 능가할 잠재력"을 가졌다고 강조하며 세간의 주목을 받았는데요. 과연 그록 3는 이러한 기대에 부응하는 혁신적인 AI일까요, 아니면 과장된 마케팅에 불과할까요? 오늘은 최신 연구 결과를 바탕으로 그록 3의 실체에 대해 깊이 파헤쳐 보겠습니다.
Elon Musk:
Elon Musk의 xAI에서 개발한 **Grok 3**는 기존 AI 모델을 능가하는 강력한 추론 능력을 가진 최신 챗봇입니다. 이 모델은 자체적으로 오류를 수정하고, 복잡한 문제 해결 능력이 뛰어나며, 다양한 분야
lilys.ai
엘론 머스크의 야심, 거대한 슈퍼컴퓨터의 탄생
그록 3의 개발 과정에서 가장 주목할 만한 부분은 바로 그 막대한 컴퓨팅 파워입니다. 엘론 머스크는 "세계에서 가장 강력한 AI 훈련 클러스터"를 가동했으며, 이는 단일 패브릭에 100,000대의 NVIDIA H100 GPU를 사용한다고 알려져 있습니다5. 이는 지금까지 AI 훈련에 동원된 가장 강력한 컴퓨팅 인프라 중 하나로 볼 수 있습니다.
AI 개발의 역사를 살펴보면, 컴퓨팅 자원은 항상 AI 발전의 핵심 동력이었습니다. 초기 모델들이 1-2 GFLOPS 성능의 단일 CPU에서 운영되었던 것에 비해, BERT-Large는 64개의 TPU v3 칩(420 TFLOPS 제공)을, GPT-3는 10,000대의 V100 GPU(28,000 TFLOPS 도달)를, GPT-4는 25,000대 이상의 NVIDIA A100 GPU(놀라운 400,000 TFLOPS 제공)를 사용했습니다1. 그리고 이제 그록 3는 이를 한 단계 더 끌어올린 것입니다.
하지만 무어의 법칙이 느려지면서 단일 칩 성능의 물리적 한계에 접근하고 있다는 사실도 주목할 필요가 있습니다1. 거대한 컴퓨팅 클러스터는 개별 칩의 한계를 보완할 수 있지만, 그러한 인프라를 유지하는 데는 천문학적인 비용이 발생합니다. GPT-4 훈련에는 1억 달러 이상이 소요된 것으로 추정되며, 각 훈련 실행은 500대의 자동차가 1년 동안 주행할 때 배출하는 CO2와 맞먹는 엄청난 에너지를 소비합니다1.
그록 3의 실제 성능은 어떨까?
빅테크 기업들 사이의 AI 경쟁이 치열해지면서, 그록 3에 대한 기대치는 하늘을 찌를 듯이 높아졌습니다. 특히 그록 3가 복잡한 문제를 해결하고 스스로 오류를 수정하는 능력이 뛰어나다는 주장이 제기되었습니다. 하지만 최근의 독립적인 연구 결과는 흥미로운 사실을 보여줍니다.
2025년 초에 진행된 다중모달 대규모 언어 모델에 대한 시각적 추론 평가 연구에 따르면, ChatGPT-o1이 전체 정확도(82.5%)와 거부 정확도(70.0%)에서 선두를 차지했으며, Gemini 2.0 Flash Experimental(70.8%)이 그 뒤를 이었습니다78. 놀랍게도 그록 3는 상당한 매개변수 수에도 불구하고 기대에 미치지 못하는 성능을 보였습니다.
이 연구의 주요 발견 중 하나는 "모델 크기만으로는 성능의 유일한 결정 요인이 아니라는 것을 그록 3의 저조한 성능이 증명한다"는 점입니다8. 그록 3는 복잡한 추론과 일관성이 필요한 작업에서 특히 낮은 성능을 보였으며, 중간 정도의 거부 정확도(0.525)는 일관적이지 않은 추론 안정성을 나타냈습니다7.
또한 심장학 분야에서의 임상적 추론을 평가한 또 다른 연구에서도 GPT-4가 전반적으로 가장 우수한 성능을 보였습니다13. 이러한 결과들은 그록 3가 아직 "지구상에서 가장 스마트한 AI"라는 주장을 입증하지 못했음을 시사합니다.
분산 AI 훈련: 엣지 컴퓨팅의 가능성
그록 3와 같은 대규모 AI 모델이 직면한 주요 도전 과제 중 하나는 막대한 컴퓨팅 요구 사항입니다. 이러한 맥락에서 분산 AI 훈련에 대한 연구가 흥미로운 대안을 제시합니다.
예를 들어, DeepSeek-v3 모델 훈련에는 각각 59.30 TFLOPS의 FP32 성능을 제공하는 2,048대의 H100 GPU가 사용되며, 총 121,446.4 TFLOPS의 컴퓨팅 용량이 필요합니다13. 만약 이 작업을 iPhone 16 시리즈와 같이 2 TFLOPS의 최대 성능을 갖춘 엣지 디바이스에 분산시킨다면, 약 60,723명의 사용자가 (이상적으로) 병렬로 작업하면 동일한 컴퓨팅 용량을 달성할 수 있습니다3.
2020년부터 2024년까지 스마트폰 칩 성능은 크게 향상되었으며, 최대 컴퓨팅 성능이 1.53 TFLOPS에서 4.95 TFLOPS로, 평균 컴퓨팅 성능이 0.48 TFLOPS에서 1.38 TFLOPS로 증가했습니다3. 이러한 추세는 엣지 컴퓨팅 파워의 급속한 확장을 보여주며, AI 애플리케이션뿐만 아니라 복잡한 AI 모델을 훈련할 잠재력도 지니고 있습니다.
그록 3의 한계와 미래 전망
그록 3는 베타 버전 상태에서 ChatGPT-o1에 대한 강력한 경쟁자로 포지셔닝되었지만, 현재까지의 연구 결과는 이 모델이 아직 기대에 부응하지 못하고 있음을 보여줍니다7. 특히 그록 3는 **비정상적으로 높은 기권률(abstention rate)**을 보였는데, 이는 올바른 옵션이 있을 때도 답변을 거부하는 경향이 있음을 나타냅니다. 이는 불확실성에 대한 지나치게 보수적인 접근 방식을 시사하며, 이로 인해 의사 결정 효율성이 저하됩니다7.
그럼에도 불구하고, 그록 3는 특정 작업에서 중간 정도의 성공을 보였으며, xAI의 지속적인 개발과 개선을 통해 향후 성능이 향상될 가능성이 있습니다. 특히 컴퓨터 칩 기술의 발전과 효율적인 모델 설계를 통해 그록 3와 같은 대규모 모델의 성능을 개선할 수 있는 여지가 있습니다.
결론: 과장된 마케팅 vs 실제 성능
그록 3는 분명 AI 분야에서 주목할 만한 시도이며, 엘론 머스크의 야심과 xAI의 기술적 역량을 보여주는 예시입니다. 그러나 현재까지의 연구 결과는 이 모델이 크기에 비해 기대에 미치지 못하는 성능을 보이고 있음을 시사합니다78.
이는 AI 개발에 있어 중요한 교훈을 제공합니다: 단순히 더 큰 모델을 만드는 것만으로는 성능 향상을 보장할 수 없으며, 효율적인 아키텍처 설계, 고품질 훈련 데이터, 세심한 최적화가 성공적인 AI 모델 개발에 결정적인 역할을 합니다.
그록 3의 사례는 AI 기술에 대한 과장된 마케팅과 실제 성능 사이의 간극을 보여주며, 소비자와a 기업이 AI 모델을 평가할 때 독립적인 벤치마크와 연구 결과를 참고하는 것의 중요성을 상기시킵니다.
앞으로 그록 3가 초기의 한계를 극복하고 진정으로 혁신적인 AI 모델로 발전할 수 있을지, 아니면 역사의 한 페이지로 남게 될지는 xAI의 지속적인 개발 노력과 시장의 반응에 달려 있을 것입니다.
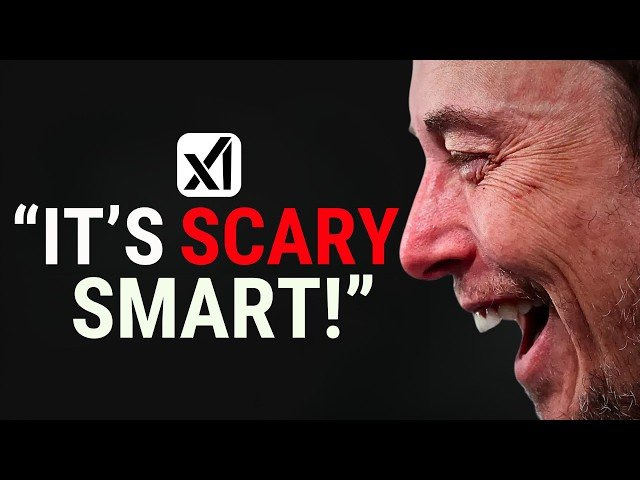
관련 태그
#그록3 #Grok3 #엘론머스크 #xAI #인공지능 #대규모언어모델 #LLM #AI개발 #AItraining #H100GPU #딥러닝 #머신러닝 #GPT4 #ChatGPT #Gemini #AIbenchmark #엣지컴퓨팅 #분산AI
Grok 3 Reality Check: Is Elon Musk's AI Revolution Hype or Truth?
Elon Musk's ambitious project to create the world's most powerful AI, Grok 3, has been generating significant buzz in the AI industry. Released on February 17, 2025, this multimodal large language model boasts an impressive 2.7 trillion parameters, making it one of the largest AI models ever developed7. Musk has emphasized that this model has "the potential to surpass all existing AI systems," attracting widespread attention. But is Grok 3 truly the revolutionary AI it claims to be, or is it just overblown marketing? Today, we'll dive deep into the reality of Grok 3 based on the latest research findings.
Elon Musk's Ambition: The Birth of a Massive Supercomputer
The most notable aspect of Grok 3's development is its immense computing power. Elon Musk has activated "the world's most powerful AI training cluster," reportedly using 100,000 NVIDIA H100 GPUs on a single fabric5. This represents one of the most powerful computing infrastructures ever deployed for AI training.
Looking at the history of AI development, computing resources have always been the key driving force behind AI advancement. While early models operated on single CPUs with 1-2 GFLOPS performance, BERT-Large used 64 TPU v3 chips (providing 420 TFLOPS), GPT-3 used 10,000 V100 GPUs (reaching 28,000 TFLOPS), and GPT-4 reportedly used over 25,000 NVIDIA A100 GPUs (delivering an astounding 400,000 TFLOPS)1. Now, Grok 3 has taken this to the next level.
However, it's worth noting that we are approaching physical limits in single-chip performance as Moore's Law slows down1. While massive computing clusters can compensate for individual chip limitations, maintaining such infrastructure incurs astronomical costs. GPT-4's training is estimated to have cost over $100 million, and each training run emits as much CO2 as 500 cars driven for a year1.
How Does Grok 3 Actually Perform?
As AI competition between big tech companies intensifies, expectations for Grok 3 have soared. There have been claims about Grok 3's superior ability to solve complex problems and self-correct errors. However, recent independent research reveals interesting facts.
According to a visual reasoning evaluation of multimodal large language models conducted in early 2025, ChatGPT-o1 led in overall accuracy (82.5%) and rejection accuracy (70.0%), followed by Gemini 2.0 Flash Experimental (70.8%)78. Surprisingly, Grok 3 underperformed despite its substantial parameter count.
One of the key findings of this study is that "Grok 3's underperformance demonstrates that model size is not the sole determinant of performance"8. Grok 3 particularly underperformed in tasks requiring complex reasoning and consistency, and its moderate rejection accuracy (0.525) indicated inconsistent reasoning stability7.
Additionally, another study evaluating clinical reasoning in cardiology found that GPT-4 showed the best overall performance13. These results suggest that Grok 3 has not yet proven the claim of being "the smartest AI on Earth."
Distributed AI Training: The Potential of Edge Computing
One of the major challenges faced by large-scale AI models like Grok 3 is their enormous computing requirements. In this context, research on distributed AI training offers an interesting alternative.
For example, training the DeepSeek-v3 model utilizes 2,048 H100 GPUs, each providing a peak FP32 performance of 59.30 TFLOPS, resulting in a total computational capacity of 121,446.4 TFLOPS13. If this workload were distributed across edge devices with a peak performance of 2 TFLOPS (e.g., mobile chips like the iPhone 16 series), approximately 60,723 users with edge devices working (ideally) in parallel would be required to match the computational capacity3.
From 2020 to 2024, smartphone chip performance has seen significant improvements, with peak computing power increasing from 1.53 TFLOPS to 4.95 TFLOPS, and average computing power rising from 0.48 TFLOPS to 1.38 TFLOPS3. This trend highlights the rapid expansion of edge computing power, which not only holds potential for AI applications but also for training complex AI models.
Limitations and Future Prospects of Grok 3
Grok 3 was positioned as a powerful competitor to ChatGPT-o1 in its Beta version, but research results so far show that the model has not yet lived up to expectations7. In particular, Grok 3 exhibited an unusually high abstention rate, indicating a tendency to reject answers even when correct options were available. This suggests an overly conservative approach to uncertainty, which undermined its decision-making effectiveness7.
Nevertheless, Grok 3 showed moderate success in specific tasks, and there is potential for improved performance through continued development and refinement by xAI. Especially through advancements in computer chip technology and efficient model design, there is room to improve the performance of large-scale models like Grok 3.
Conclusion: Exaggerated Marketing vs. Actual Performance
Grok 3 is undoubtedly a notable attempt in the field of AI and demonstrates Elon Musk's ambition and xAI's technical capabilities. However, research results to date suggest that this model is underperforming relative to its size78.
This provides an important lesson in AI development: Simply creating larger models does not guarantee performance improvements. Efficient architecture design, high-quality training data, and careful optimization play crucial roles in successful AI model development.
The case of Grok 3 illustrates the gap between exaggerated marketing of AI technology and actual performance, reminding consumers and businesses of the importance of consulting independent benchmarks and research results when evaluating AI models.
Whether Grok 3 will overcome its initial limitations and evolve into a truly innovative AI model or remain as a page in history will depend on xAI's continued development efforts and market response.
Related Tags
#Grok3 #ElonMusk #xAI #ArtificialIntelligence #LargeLanguageModel #LLM #AIDevelopment #AItraining #H100GPU #DeepLearning #MachineLearning #GPT4 #ChatGPT #Gemini #AIbenchmark #EdgeComputing #DistributedAI
Citations:
- https://arxiv.org/pdf/2503.08223.pdf
- https://arxiv.org/html/2502.19261
- https://arxiv.org/html/2503.08223v1
- https://arxiv.org/html/2406.01566v2
- https://arxiv.org/pdf/2409.19913.pdf
- https://arxiv.org/html/2410.04466v2
- https://arxiv.org/pdf/2502.16428.pdf
- https://arxiv.org/html/2502.16428v1
- https://www.semanticscholar.org/paper/927df50dd20af3dad169027fa8006fd53d48f119
- https://arxiv.org/abs/2502.16428
- https://www.semanticscholar.org/paper/bf11f518b28734225d25382e3babcdfa64b69dde
- https://www.semanticscholar.org/paper/02e003d4cb4a67d46bd2f555c6c80ecc2af3c210
- https://www.semanticscholar.org/paper/770e1902559a33eb38953c462d90502ccae35c09
- https://www.semanticscholar.org/paper/c63dbe42225bbbac1f8462349a480966e78e8ad2
'이슈 > AI' 카테고리의 다른 글
AI 시대, 검색의 새로운 패러다임: 라이너와 함께 탐험하는 정보의 대항해 (3) | 2025.03.20 |
---|---|
AI 마스터의 노트앱과 전율적인 정보 검색 방법 배우기 (2) | 2025.03.20 |
🌟 AI 리더십의 미래: 미국의 '풀스택 전략'과 글로벌 경쟁 구도 (0) | 2025.03.19 |
리테일 테크의 본질: 기술이 아닌 솔루션을 고민하라 (0) | 2025.03.19 |
NVIDIA GTC와 FTC 규제 방향: 기술 시장의 현재와 미래 전망 (0) | 2025.03.19 |